Revolutionizing Healthcare: The Importance of Datasets for Machine Learning
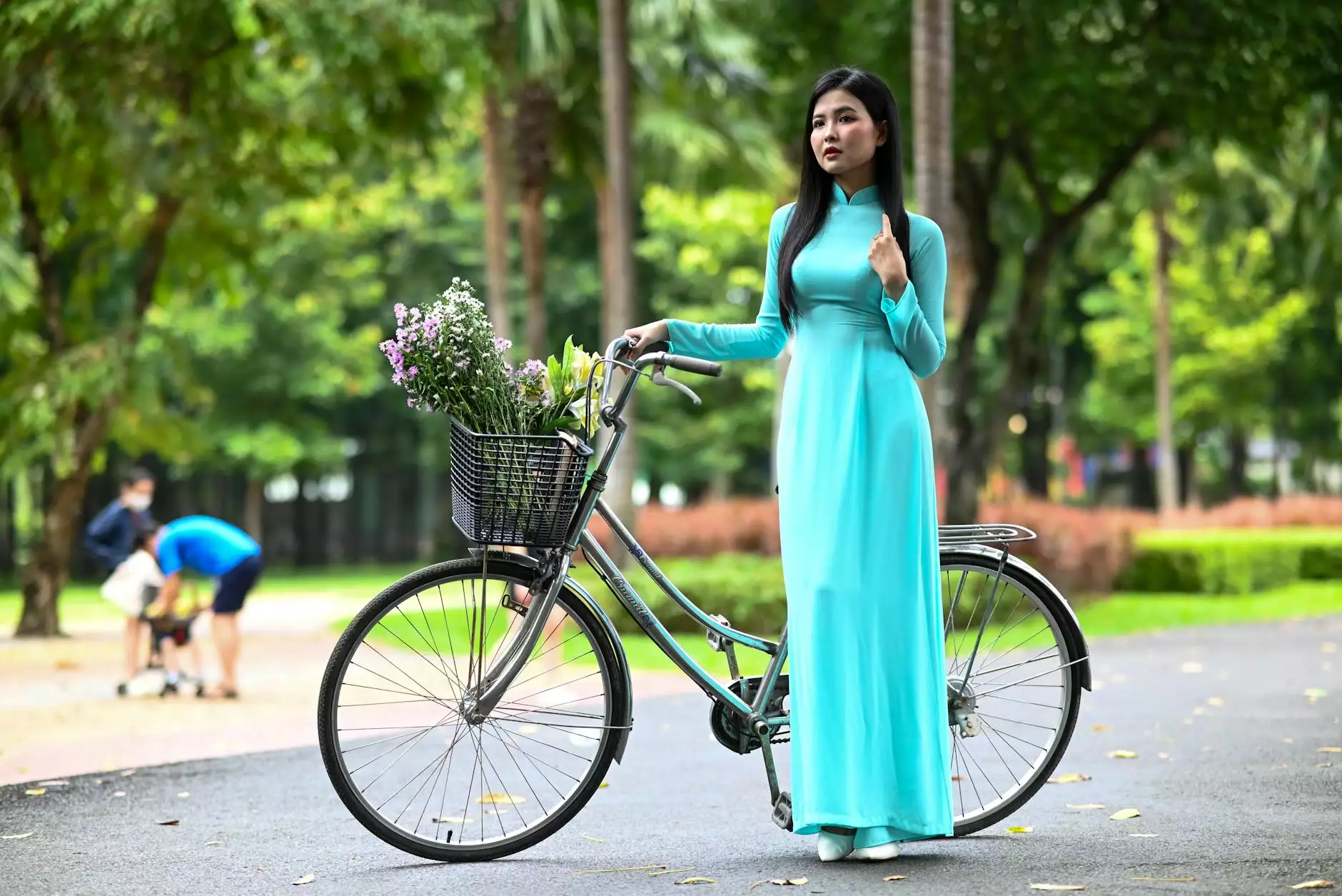
In the competitive landscape of modern business, particularly in the healthcare sector, the integration of technology and data has become a cornerstone for success. One of the most promising advancements in this domain is the utilization of healthcare datasets for machine learning. This article delves deep into how these datasets are transforming healthcare practices while enhancing business productivity and patient care.
The Role of Machine Learning in Healthcare
Machine learning (ML) is a subset of artificial intelligence (AI) that enables computers to learn from and make predictions based on data. In healthcare, ML is increasingly being applied to solve complex problems ranging from patient diagnosis to treatment personalization. This close relationship between ML and healthcare sets the stage for significant advancements in treatment outcomes and operational efficiency.
Understanding Healthcare Datasets
Healthcare datasets are large collections of health information that include various formats such as structured records, unstructured notes, images, and genomic information. These datasets can be derived from different sources:
- EHRs (Electronic Health Records): Comprehensive patient history and treatment information.
- Claims Data: Information regarding patient insurance claims, providing insights into treatment patterns and costs.
- Clinical Trials: Data from trials that assess the efficacy and safety of new drugs and treatments.
- Genomic Data: Information related to genetic Make-up, increasingly important in personalized medicine.
- Wearable Devices: Data collected from devices that monitor health metrics, providing real-time insights into patient conditions.
The Significance of Quality Datasets
The quality of healthcare datasets for machine learning is paramount. Poor quality data can lead to inaccurate predictions, misguided treatments, and ultimately, poor patient outcomes. Quality datasets possess several characteristics:
- Accuracy: Data must reflect true patient conditions without errors.
- Completeness: All necessary data points should be captured to ensure comprehensive analysis.
- Consistency: Information should be the same across various data sources and formats.
- Timeliness: Data should be collected in real time to provide the most up-to-date insights.
- Relevance: Datasets should specifically cater to the healthcare domain to ensure meaningful analysis.
Applications of Machine Learning in Healthcare
The applications of machine learning, powered by healthcare datasets, can significantly enhance business operations and patient care:
1. Predictive Analytics
Machine learning models can analyze historical data to predict future outcomes. For instance, hospitals can use ML to forecast patient admission rates, helping them manage resources efficiently.
2. Personalized Treatment Plans
With insights drawn from comprehensive healthcare datasets, ML algorithms can assist in creating individualized treatment plans. This ensures that each patient receives care tailored to their unique health profile.
3. Early Detection of Diseases
By analyzing patterns in healthcare datasets, machine learning can help in the early detection of diseases, such as diabetes or cancer, which is crucial for improving treatment success rates.
4. Operational Efficiency
Healthcare organizations can streamline their operations by identifying inefficiencies through data analysis, thus reducing costs and improving service delivery.
5. Drug Development
In pharmaceutical companies, machine learning models can expedite the drug discovery process by predicting which compounds may be effective against specific diseases based on existing datasets.
Challenges in Utilizing Healthcare Datasets
While the potential benefits of using healthcare datasets for machine learning are vast, certain challenges must be addressed:
1. Data Privacy Concerns
With data breaches increasingly common, maintaining patient confidentiality is imperative. Regulations such as HIPAA in the United States ensure that healthcare data is handled responsibly.
2. Data Integration
Integrating data from various sources can be complex and time-consuming. Ensuring compatibility and coherence among different types of data is critical for effective analysis.
3. Need for Skilled Professionals
The effective analysis of healthcare datasets requires skilled data scientists and healthcare professionals who can interpret the results meaningfully.
4. Evolving Technologies
As technology advances, staying updated with the latest machine learning techniques and tools becomes essential. Organizations must invest in continual learning and development.
Case Studies: Success Stories in the Healthcare Sector
Several healthcare organizations have successfully integrated machine learning through robust datasets, yielding impressive results:
Case Study 1: Predictive Analytics in Hospital Management
A leading hospital in New York utilized machine learning to forecast patient discharge times, allowing them to optimize bed allocation. This improved patient flow and reduced waiting times significantly.
Case Study 2: Disease Prediction Models
A renowned healthcare startup developed an ML-driven model that analyzes patient data to predict the onset of diabetes. By providing early intervention, the startup contributed to improved patient health outcomes.
Case Study 3: Genomic Data in Cancer Treatment
By employing machine learning on genomic datasets, a pharmaceutical company was able to identify biomarkers associated with specific cancers, leading to more targeted therapies.
The Future of Machine Learning in Healthcare
The future of machine learning in healthcare is bright, with continuous advancements expected in technology and data management. Key trends to watch include:
- Increased Use of AI: AI will increasingly play a role in interpreting complex datasets, significantly enhancing precision in diagnosis and treatment.
- Telehealth Integration: The rise of telehealth services will necessitate the continuous collection and analysis of healthcare data to improve virtual care.
- Blockchain for Data Security: Utilizing blockchain technology can enhance data security and integrity while ensuring privacy remains intact.
- Collaborative Data Sharing: Breaking down data silos through collaborative initiatives will provide richer datasets for more robust machine learning applications.
Conclusion
In conclusion, the integration of healthcare datasets for machine learning is a transformative force in the healthcare industry. By harnessing the power of data through effective machine learning applications, healthcare professionals can offer more personalized care while significantly optimizing operational efficiencies. As the industry continues to evolve, embracing these advancements will undoubtedly pave the way for improved health outcomes and sustainable business practices.
For businesses in the healthcare sector, the key takeaway is clear: investing in quality data and machine learning technology can lead to remarkable improvements in patient care and operational success. As we move forward, the synergy between data science and healthcare holds vast potential that remains to be fully realized.